Our methods
Concurrent
TMS-fMRI
Information flow analysis
Comparison of EEG systems
Errors
analysis
Decoding of deicison making
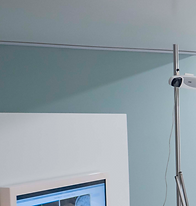
Concurrent TMS-fMRI
We are currently setting up a new TMS-fMRI system at the CBU, using an MR compatible TMS system combined with two MR surface coils. These are placed between the TMS coil and the participant’s scalp, improving signal directly beneath the TMS coil. We hope to investigate the direct effects of TMS on the brain during different tasks and stimulation protocols.
Key publications: Jackson 2020
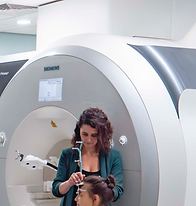
Information Flow Analysis
We have developed a new information flow analysis (IFA) method that allows us to track the exchange of information between brain regions. Further developments will use the IFA methods to study not only whether and when information is exchanged but also what that information is, and the relationship between information exchange and standard connectivity metrics (e.g., phase synchrony).
Watch: video of Alex discussing IFA approaches here
Key publications: Goddard et al 2016, Goddard et al 2018
Comparison of EEG systems
Recently, portable, low-cost EEG systems have become available offering exciting possibilities for more convenient testing. We are validating one such system, the Emotiv EPOC+, against traditional research-grade systems to find out whether this fast setup and easy to use headset can be used to examine language processing in children with autism.
Key Publications: Petit et al 2020 JLSHR
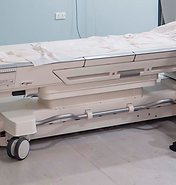
Errors analysis
Key insights into how information coding in the brain supports behaviour could come from examining what information is coded when people make mistakes. Typically this is hard to do because participants make few mistaked and decoding analyses require many trials. However, the requirement can be met by training classifiers using the plentiful data from correctly performed trials.
Key Publications: Woolgar et al 2019, BioRxiv
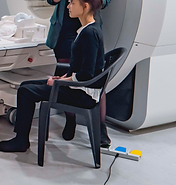

Decoding Decision-Making
We explore the extent to which features of multivariate pattern analysis classifiers reflect latent variables of classic decision making models during perceptual decision-making. For example, we study how classifier hyperplanes relate to cognitive decision boundaries, or classification accuracy reflects perceptual certainty. These relationships predict choice behaviour and as such, we hope to identify neural activity that is appropriately formatted for the brain to use in guiding behaviour.
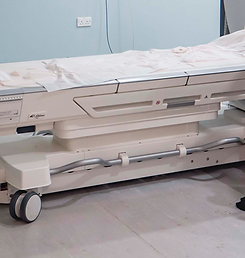